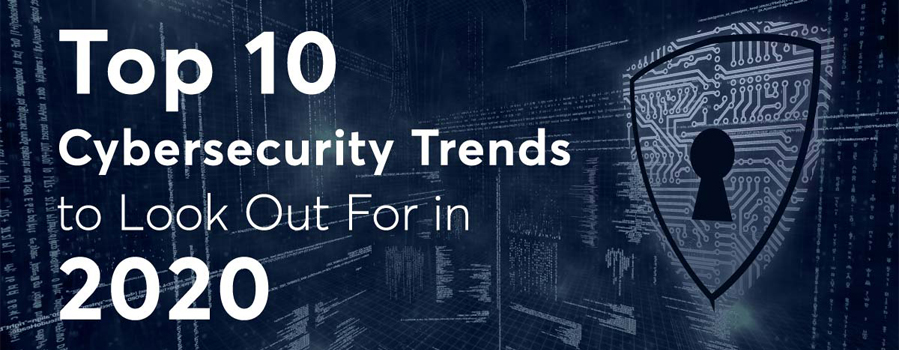
Top 10 Cybersecurity Trends to Look Out For in 2020
26th February 2020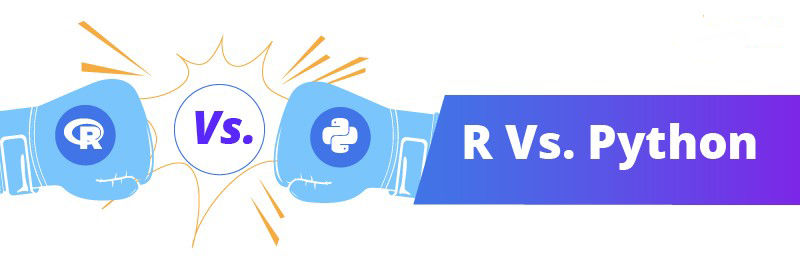
R vs Python
2nd March 2020In this 21st century that revolves around the enormously growing data, it has become a necessity for humans to create powerful processing machines. These machines should provide automation for processing large amounts of data. To build all these systems, we require professionals such as Machine Learning Engineer and Data Scientist. This blog is entirely focused on the concept of Machine Learning Engineer vs Data Scientist, and it’s a career comparison study.
“Knowledge is power. Data is the new currency. Data Science is both,” thus the saying goes! So, where Machine Learning (ML) comes in? Well, it is like this – without ML, you cannot leverage automation. Although the data would be the same, its value wouldn’t be that much. Before elucidating the Machine Learning Engineer vs. Data Scientist topic in detail, let us quickly glance through some hard facts:
Machine Learning vs. Data Science in terms of Growth rate and market cap.
Data Science | Machine Learning | |
Growth Rate | 30.0% per year | 42.8% per year |
Market Value | US$56 billion | US$132 billion |
Top 5 Companies Hiring | Google, Facebook, Amazon, Oracle, & Netflix | Google, Facebook, Amazon, Oracle, & Netflix |
What is Data Science?
Before understanding Machine Learning in this ‘Machine Learning Engineer vs Data Scientist’ blog, we will go through an introduction to Data Science and the skills required to become a Data Scientist. Putting it in a simple way, Data Science is the study of data. It involves the visualization and analysis of data collected from multiple sources. These visualizations help in gaining useful insights from the data. If you want to learn Data Science and Machine Learning together then you can sign up for Data Science Architect Master’s Course.
Data Science uses the data for competitive analysis and knowing the current market trends. Also, it helps us look into the patterns and trends in the dataset, thereby identifying the scope for improvements in business processes. This, in turn, helps in boosting revenue generation.
Why do we use Data Science?
According to Forbes, the amount of data generated per day is 2.5 quintillion bytes, and it is still growing at a high rate. With such a huge amount of data in-store, it becomes necessary for organizations to make use of this voluminous data to yield huge benefits and compete in the market. This is where Data Science comes into the picture.
Data Science consists of various tools and techniques that help in understanding data easily. By having a thorough knowledge and understanding of business data, we can create a better-optimized business model.
Let’s understand the use of Data Science with the help of a use case.
Interested in learning Data Science? Click here to learn more in this Data Science Training in Bangalore!
Data Science Use Case: Product Recommendation Systems
Today, there are millions of people who buy products online instead of visiting a store. This is due to the affordable prices that online websites offer to their customers. On the backstage, while shopping online, the customers provide their Internet surfing data. This surfing data consists of the following:
1. Data of the visited sites
2. Products clicked
3. Products added to the cart
4. Stay time on websites or on a particular product
Companies use these parameters to build automated software using Machine Learning. These software help predict whether a customer will buy a product or not. Also, if there are greater chances of customers buying a particular product, then it recommends the product to those customers. These recommendations reflect on the customers’ Facebook accounts and other websites they use. This is a type of smart and efficient marketing. So, Data Science assists a lot in generating smart business processes by analyzing and visualizing the data.
Now, we will look into the skills required to become a Data Scientist.
Data Scientist Skills
The field of Data Science requires certain skills to master it. The skillset required to become a Data Scientist comprises the following:
- Python or R programming language
- Mathematics (statistics and probability)
- Data visualization tools (Tableau or Power BI)
- Problem-solving skills
- MS Excel for data analysis
- Machine Learning algorithms
Data Scientist Salary and Scope
According to Naukri.com, the number of job postings for a Data Scientist is more than 8,000 in January 2020 in India and, in the United States, the number is around 15,000. This huge number shows us a wide scope in the field of Data Science. Consequently, the average salary paid to a Data Scientist in India is ₹625,000 and, in the United States, it is US$110,000. Let us look at the salary on the basis of experience:
Experience | Salary in the United States | Salary in India |
0-1 Years | US$79,259 | ₹625,489 |
1-3 Years | US$95,250 | ₹856,250 |
3-5 Years | US$115,358 | ₹1,356,500 |
5+ Years | US$130,785 | ₹2,250,825 |
The below graph shows the job trends of a Data Scientist from 2004 to the present:
By these stats, we can infer that there is a bright future for all aspiring Data Scientists.
Now, let’s look at the number of job postings for a Data Scientist according to some the regions:
Location | Number of Jobs |
Bangalore | 3,209 |
New York | 2,204 |
London | 3,824 |
Berlin | 1,825 |
California | 5,365 |
Seattle | 1,145 |
Now, let’s move ahead and understand what Machine Learning is.
What is Machine Learning?
Machine Learning is a branch of Artificial Intelligence. It deals with the creation of automated machines/models that learn by experience. We use various algorithms to build these Machine Learning models. Then, we expose the automated machines/models to different varieties of datasets for training. This helps in making the machines/models perfect for working in a real-time environment, by making them learn on their own. It is all a Machine Learning Engineer that makes this possible. Now, let us have a glance at why we use Machine Learning.
Learn Machine Learning from experts, click here to more in this Machine Learning Training in London!
Why do we use Machine Learning?
In earlier days, human beings used to store data manually. This is because the data generation sources were very less those days. On the other hand, we all know that now we are living in a data-centric world where the amount of data generated per day is in quintillion bytes. However, we can use this data to make a huge difference in profits. It is to make this possible, we use Machine Learning. We build systems using Machine Learning that help in smart automation. This includes digital marketing, recommendation systems, and power processing.
As discussed in the use case of product recommendation systems, a Data Scientist visualizes data. These visualizations give insights into the data, and the reports are handed over to a Machine Learning Engineer. Then, the ML Engineer understands this data thoroughly. After that, he/she decides about the appropriate algorithm that should be used for building an efficient automated system.
Now, in this ‘Machine learning Engineer vs Data Scientist’ blog, let’s see the major companies who use Machine Learning.
1. Netflix: Netflix uses a recommendation system to recommend relevant TV shows and movies to its viewers.
2. Flipkart: Flipkart uses it for suggesting products to its potential customers. They recommend products on their websites as well as advertise it on other websites that you visit.
3. Grammarly: Grammarly uses Machine Learning for the analysis of the text so that it can suggest changes and correct errors.
4. Google: With the help of Machine Learning, Google provides a personalized experience to its users. All the applications of Google such as Google Search, Google Maps, and Google Translate use Machine Learning.
Now, coming to the major difference between Machine Learning Engineer and Data Scientist, it lies in the usage of Deep Learning concepts. Data Scientists know only the algorithms of Machine Learning. They assist ML Engineers to build automated software. But, ML Engineers implement Machine Learning, as well as Deep Learning, algorithms to make accurate working models.
Machine Learning Engineer Skills
To become a successful Machine Learning Engineer, we have to master the below-mentioned skills:
1. Mathematics (linear algebra, calculus, statistics, and probability)
2. Problem-solving skills
3. Software engineering and system design
4. Data modeling and evaluation
5. Reinforcement learning
6. Natural Language Processing
7. Machine Learning algorithms and Deep Neural Networks
Machine Learning Engineer Salary and Scope
In 2020, the demand for a Machine Learning Engineer is at a high rise. This is because we need automation for smart and efficient business processes.
According to Indeed, there are 8,000+ vacant jobs available for a skilled Machine Learning Engineer. Industries are interested in paying a decent package for the right skill set.
The average salary of a Machine Learning Engineer is more than that of a Data Scientist. In the United States, it is around US$125,000 and, in India, it is ₹875,000. This salary structure is more than sufficient to decide for a bright career as a Machine Learning Engineer.
Experience | Salary in the United States | Salary in India |
0-1 Years | US$85,256 | ₹650,000 |
1-3 Years | US$105,351 | ₹975,023 |
3-5 Years | US$118,054 | ₹1,525,485 |
5+ Years | US$135,096 | ₹2,450,825 |
Now, in this blog on ‘Machine Learning Engineer vs Data Scientist,’ let us look into the graph of job trends for a Machine Learning Engineer.
Now, let’s check out the number of job postings for a Machine Learning Engineer according to some regions:
Location | Number of Jobs |
Bangalore | 4,374 |
New York | 4,415 |
London | 6,954 |
Berlin | 1,433 |
California | 13,025 |
Seattle | 5,185 |
There are various sectors where Data Science and Machine Learning are applicable. Below is the list of sectors and top companies using Data Science and Machine Learning.
Application | Data Science | Machine Learning |
Healthcare | BrainWeb, OASIS, fastMRI, etc | Catalyst, Healthcare.ai, etc. |
Banking & Finance | Citi Bank, Bank of America, ICICI, etc. | US Bank, Citi Bank, Bank of NY, etc. |
E-commerce | Flipkart, Amazon, Healthkart, etc. | Flipkart, Amazon, Healthkart, etc. |
Automobile | Tesla, drive.ai, NuTonomy, etc. | Tesla, AutoX, Optimus Ride, etc. |
Bioinformatics | Biomax Informatics, Qlucore, etc. | Metalife, Biodi |
In this blog, we tried to understand the difference between Machine Learning and Data Scientist. Also, we looked at some use cases to understand why we use Data Science and Machine Learning in real life.
This is all about ‘Machine Learning Engineer vs Data Scientist.’