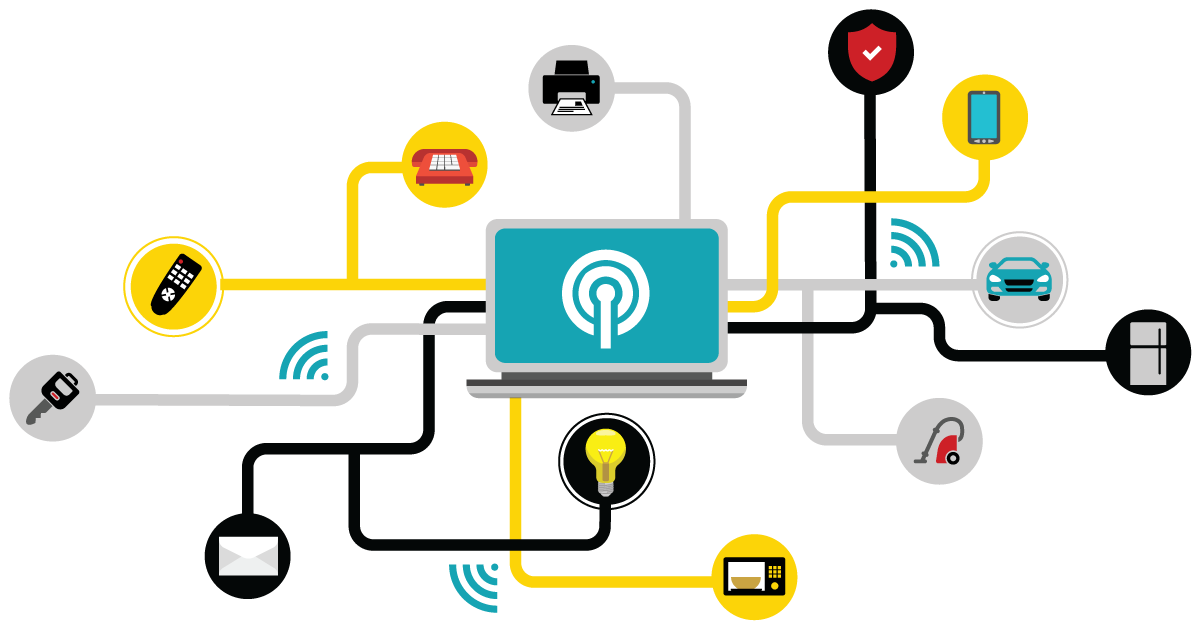
Major Infrastructure Challenges in IoT Development
2nd January 2020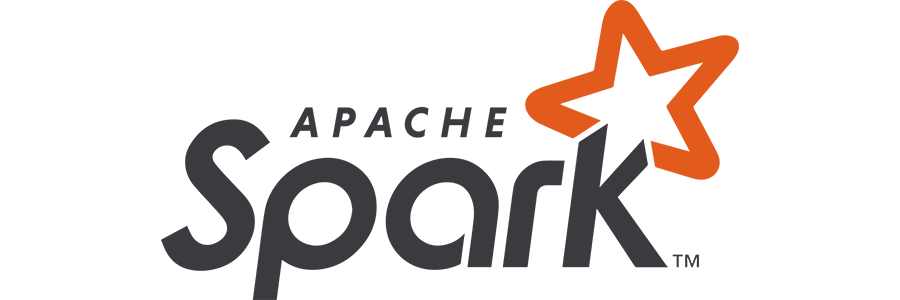
What is Apache Spark?
6th January 2020Artificial intelligence (AI) is the simulation of human intelligence processes by machines, especially computer systems. Specific applications of AI include expert systems, natural language processing (NLP), speech recognition and machine vision.
AI programming focuses on three cognitive skills: learning, reasoning and self-correction.
Learning processes. This aspect of AI programming focuses on acquiring data and creating rules for how to turn the data into actionable information. The rules, which are called algorithms, provide computing devices with step-by-step instructions for how to complete a specific task.
Reasoning processes. This aspect of AI programming focuses on choosing the right algorithm to reach a desired outcome.
Self-correction processes. This aspect of AI programming is designed to continually fine-tune algorithms and ensure they provide the most accurate results possible.
Advantages and disadvantages of artificial intelligence
Artificial neural networks and deep learning artificial intelligence technologies are quickly evolving, primarily because AI processes large amounts of data much faster and makes predictions more accurately than humanly possible. While the huge volume of data that’s being created on a daily basis would bury a human researcher, AI applications that use machine learning can take that data and quickly turn it into actionable information. As of this writing, the primary disadvantage of using AI is that it is expensive to process the large amounts of data that AI programming requires.
Strong AI vs. Weak AI
AI can be categorized as either weak or strong. Weak AI, also known as narrow AI, is an AI system that is designed and trained to complete a specific task. Industrial robots and virtual personal assistants, such as Apple’s Siri, use weak AI.
Strong AI, also known as artificial general intelligence (AGI), describes programming that can replicate human cognitive abilities. When presented with an unfamiliar task, a strong AI system can use fuzzy logic to apply knowledge from one domain to another and find a solution autonomously. In theory, a strong AI program should be able to pass both a Turing test and the Chinese room test.
Augmented intelligence vs. artificial intelligence
Some industry experts believe that the term artificial intelligence is too closely linked to popular culture, and this has caused the general public to have improbable expectations about how AI will change the workplace and life in general. Some researchers and marketers hope the label augmented intelligence, which has a more neutral connotation, will help people understand that most implementations of AI will be weak and simply improve products and services. The concept of the Singularity and a world where the application of superintelligence to humans or human problems — including poverty, disease and mortality — still falls within the realm of science fiction.
Ethical use of artificial intelligence
While AI tools present a range of new functionality for businesses, the use of artificial intelligence also raises ethical questions because, for better or worse, an AI system will reinforce what it has already learned.
This can be problematic because machine learning algorithms, which underpin many of the most advanced AI tools, are only as smart as the data they are given in training. Because a human being selects what data is used to train an AI program, the potential for machine learning bias is inherent and must be monitored closely.
Anyone looking to use machine learning as part of real-world, in-production systems needs to factor ethics into their AI training processes and strive to avoid bias. This is especially true when using AI algorithms that are inherently unexplainable in deep learning and generative adversarial network (GAN) applications.
Explainability and artificial intelligence
Explainability is a potential stumbling block to using AI in industries that operate under strict regulatory compliance requirements. For example, financial institutions in the United States operate under regulations that require them to explain their credit-issuing decisions. When a decision to refuse credit is made by AI programming, however, it can be difficult to explain how the decision was arrived at because the AI tools used to make such decisions operate by teasing out subtle correlations between thousands of variables. When the decision-making process cannot be explained, the program may be referred to as black box AI.
Components of AI
As the hype around AI has accelerated, vendors have been scrambling to promote how their products and services use AI. Often what they refer to as AI is simply one component of AI, such as machine learning. AI requires a foundation of specialized hardware and software for writing and training machine learning algorithms. No one programming language is synonymous with AI, but a few, including Python and C, have set themselves apart.
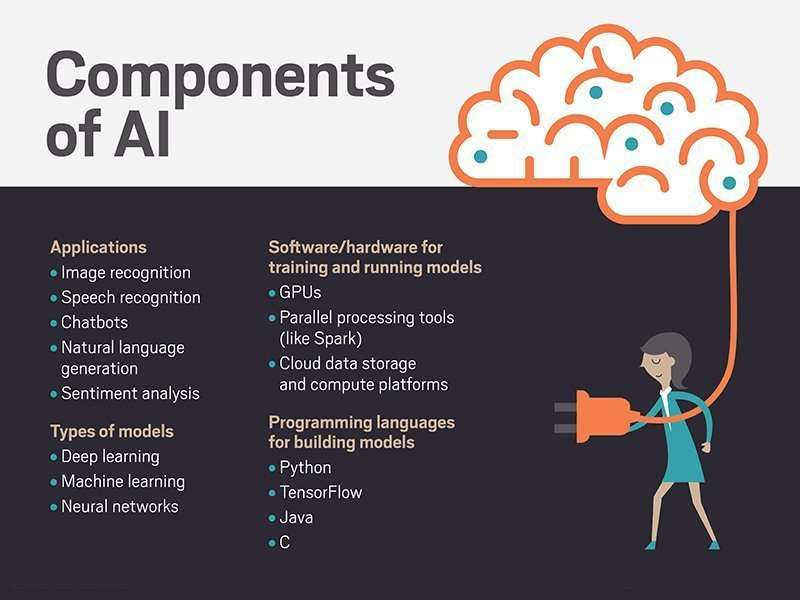
AI as a service (AIaaS)
Because hardware, software and staffing costs for AI can be expensive, many vendors are including AI components in their standard offerings or providing access to artificial intelligence as a service (AIaaS) platforms. AIaaS allows individuals and companies to experiment with AI for various business purposes and sample multiple platforms before making a commitment.
Popular AI cloud offerings include the following:
- Amazon AI
- IBM Watson Assistant
- Microsoft Cognitive Services
- Google AI
Four types of artificial intelligence
Arend Hintze, an assistant professor of integrative biology and computer science and engineering at Michigan State University, categorized AI into four types, beginning with the intelligent systems that exist today to sentient systems, which do not yet exist. His categories are as follows:
- Type 1: Reactive machines. These AI systems have no memory and are task specific. An example is Deep Blue, the IBM chess program that beat Garry Kasparov in the 1990s. Deep Blue can identify pieces on the chessboard and make predictions, but because it has no memory, it cannot use past experiences to inform future ones.
- Type 2: Limited memory. These AI systems have memory, so they can use past experiences to inform future decisions. Some of the decision-making functions in self-driving cars are designed this way.
- Type 3: Theory of mind. Theory of mind is a psychology term. When applied to AI, it means that the system would understand emotions. This type of AI will be able to infer intentions and predict behavior when it becomes available.
- Type 4: Self-awareness. In this category, AI systems have a sense of self, which gives them consciousness. Machines with self-awareness understand their own current state. This type of AI does not yet exist.
Cognitive computing and AI
The terms AI and cognitive computing are sometimes used interchangeably, but, generally speaking, the label AI is used in reference to products and services that automate tasks, while the label cognitive computing is used in reference to products and services that augment human thought processes.
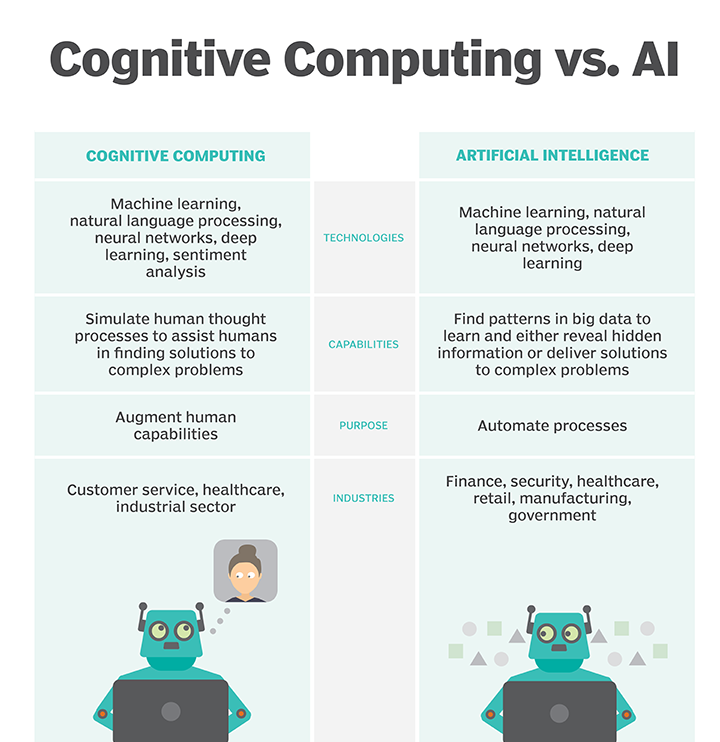
Examples of AI technology
AI is incorporated into a variety of different types of technology. Here are seven examples:
- Automation. This makes a system or process function automatically. For example, robotic process automation (RPA) can be programmed to perform high-volume, repeatable tasks that humans normally performed. RPA is different from IT automation in that it can adapt to changing circumstances.
- Machine learning. This is the science of getting a computer to act without programming. Deep learning is a subset of machine learning that, in very simple terms, can be thought of as the automation of predictive analytics. There are three types of machine learning algorithms:
- Supervised learning. Data sets are labeled so that patterns can be detected and used to label new data sets.
- Unsupervised learning. Data sets aren’t labeled and are sorted according to similarities or differences.
- Reinforcement learning. Data sets aren’t labeled but, after performing an action or several actions, the AI system is given feedback.
- Machine vision. This is the science of allowing computers to see. This technology captures and analyzes visual information using a camera, analog-to-digital conversion and digital signal processing. It is often compared to human eyesight, but machine vision isn’t bound by biology and can be programmed to see through walls, for example. It is used in a range of applications from signature identification to medical image analysis. Computer vision, which is focused on machine-based image processing, is often conflated with machine vision.
- Natural language processing. This is processing of human — and not computer — language by a computer program. One of the older and best-known examples of NLP is spam detection, which looks at the subject line and the text of an email and decides if it’s junk. Current approaches to NLP are based on machine learning. NLP tasks include text translation, sentiment analysis and speech recognition.
- Robotics. This field of engineering focuses on the design and manufacturing of robots. Robots are often used to perform tasks that are difficult for humans to perform or perform consistently. They are used in assembly lines for car production or by NASA to move large objects in space. Researchers are also using machine learning to build robots that can interact in social settings.
- Self-driving cars. These use a combination of computer vision, image recognition and deep learning to build automated skill at piloting a vehicle while staying in a given lane and avoiding unexpected obstructions, such as pedestrians.
AI applications
Artificial intelligence has made its way into a wide variety of markets. Here are six examples:
- AI in healthcare. The biggest bets are on improving patient outcomes and reducing costs. Companies are applying machine learning to make better and faster diagnoses than humans. One of the best-known healthcare technologies is IBM Watson. It understands natural language and can respond to questions asked of it. The system mines patient data and other available data sources to form a hypothesis, which it then presents with a confidence scoring schema. Other AI applications include chatbots, a computer program used online to answer questions and assist customers, to help schedule follow-up appointments or aid patients through the billing process and virtual health assistants that provide basic medical feedback.
- AI in business. Robotic process automation is being applied to highly repetitive tasks normally performed by humans. Machine learning algorithms are being integrated into analytics and CRM platforms to uncover information on how to better serve customers. Chatbots have been incorporated into websites to provide immediate service to customers. Automation of job positions has also become a talking point among academics and IT analysts.
- AI in education. AI can automate grading, giving educators more time. It can assess students and adapt to their needs, helping them work at their own pace. AI tutors can provide additional support to students, ensuring they stay on track. And it could change where and how students learn, perhaps even replacing some teachers.
- AI in finance. AI in personal finance applications, such as Intuit’s Mint or TurboTax, is disrupting financial institutions. Applications such as these collect personal data and provide financial advice. Other programs, such as IBM Watson, have been applied to the process of buying a home. Today, artificial intelligence software performs much of the trading on Wall Street.
- AI in law. The discovery process — sifting through documents — in law is often overwhelming for humans. Automating this process is a more efficient use of time. Startups are also building question-and-answer computer assistants that can sift programmed-to-answer questions by examining the taxonomy and ontology associated with a database.
- AI in manufacturing. This is an area that has been at the forefront of incorporating robots into the workflow. Industrial robots used to perform single tasks and were separated from human workers, but as the technology advanced that changed.
- AI in banking. Banks have been finding good results in using chatbots to make their customers aware of additional services and offerings. They are also using AI to improve decision-making for making loans, setting credit limits and identifying investment opportunities.
AI in security
AIand machine learning are at the top of the buzzword list security vendors are using today to differentiate their offerings. Those terms also represent truly viable technologies. Artificial intelligence and machine learning in cybersecurity products are adding real value for the security teams looking for ways to identify attacks, malware and other threats.
Organizations today use machine learning in security information and event management (SIEM) software and related areas to detect anomalies and identify suspicious activities that indicate threats. By analyzing data and using logic to identify similarities to known malicious code, AI can provide alerts to new and emerging attacks much sooner than human employees and previous technology iterations.
As a result, AI security technology both dramatically lowers the number of false positives and gives organizations more time to counteract real threats before damage is done. The maturing technology is playing a big role in helping organizations fight off cyberattacks.
Regulation of AI technology
Despite potential risks, there are currently few regulations governing the use of AI tools, and where laws do exist, they typically pertain to AI indirectly. For example, as previously mentioned, United States Fair Lending regulations require financial institutions to explain credit decisions to potential customers. This limits the extent to which lenders can use deep learning algorithms, which by their nature are opaque and lack explainability.
The Europe Union’s GDPR puts strict limits on how enterprises can use consumer data, which impedes the training and functionality of many consumer-facing AI applications.
In 2016, the National Science and Technology Council issued a report examining the potential role governmental regulation might play in AI development, but it did not recommend specific legislation be considered. Since that time the issue has received little attention from lawmakers.